Given the growing interest in Artificial Intelligence (AI), I am taking a deep dive into the topic. This exploration is in collaboration with Zone7, who are adding to the discussions from an injury risk and performance modelling perspective. So far, we have defined important terminology and explored data quantity and quality.
As we move through the data pipeline to analysis, there was one term that kept coming up: Context. Practitioners ask:
“We always talk about the context of data. How does AI take account of context in the data?”
So why is context at the forefront of practitioner minds? And, can AI include relevant context to make it more usable? In this post, we’ll explore these questions.
Why Context is Key
Implementing sports science should always incorporate a critical appraisal of the context. Textbooks present theories in an unequivocal manner, yet the application of scientific theory requires a wider appreciation of the circumstance.
Performance and injury are complex constructs. Contextual factors influence the web of determinants that drive their emergent outcomes (Bittencourt et al., 2016). Some constraints, like the rules of the game, are (mostly) fixed. Meanwhile, there are a multitude of other factors, such as schedules, player availability, and fitness-fatigue relationships, that are in continuous flux.
The sport’s demands, playing positions, and the individual athlete are each contextual foundations. Additionally, we are required to vary our perspective to the changing contextual landscape, particularly across different times of the season. Let’s illustrate these factors with examples.
Context starts with the sport and positional demands
I’ve previously discussed the challenge of updating my own practitioner lens to transition from football to ice hockey, and then later to American football. Training theory remains the same, but the context within which it is applied is grossly different.
Ice hockey is played on ice (obviously!)… But how does this influence load monitoring? What does the difference in ground reaction forces (ice v land) mean for external load tracking? How do we account for gliding, whereby athletes are moving over the ice without physiological cost to that movement?
Meanwhile, American football presents the most diverse, within-squad load measurement and management challenge of any team sport. The positional demands greatly differ. Consider, for example, the varied physical requirements of a quarterback, a lineman, a wide receiver, a kicker, and a long snapper, to name a few positions. In addition, “Special Teams” demands can be wildly different compared to a player’s primary role on Offense/Defense.
We can undoubtedly learn much from other sports. Yet, the unique context of each requires translation of processes, rather than transplanting them. As we illustrated in our Sports Medicine Open review of tracking systems in team sports, sport- and position-specific analyses are required to provide meaningful insights into athlete management.
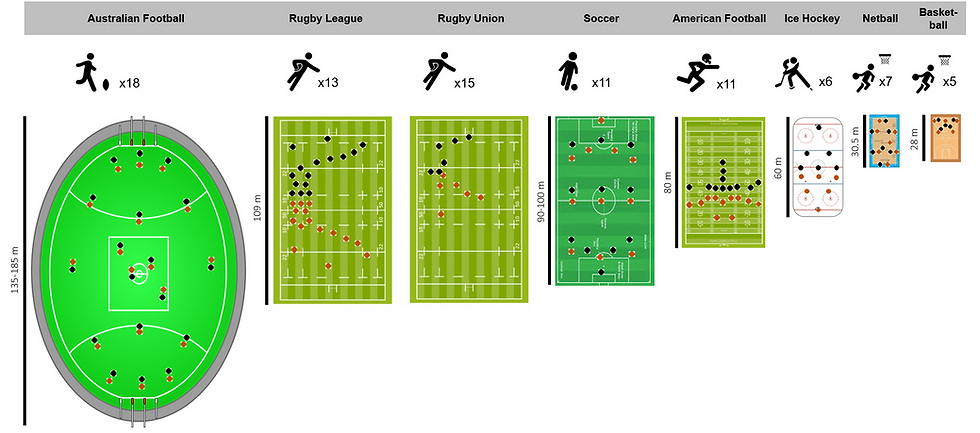
How can AI take into account the contextual demands across the different sports and playing positions?
For a data science approach to make any sense in sports performance, it must ‘speak’ the language of the specific sport. This is all about injecting the “context” discussed above into the predictive models. Another way of thinking about this is to ‘digitise’ some of the sport-specific context.
Here are some questions that Zone7 often seek to answer when diving into a new sport or environment:
Injury epidemiology per sport varies dramatically and is influenced in different ways by the contact and physical demands of the sport. What are common injuries and mechanisms in this sport? Do they interact in subtle ways?
Micro seasonality - as an example, NBA, like ice hockey and baseball (MLB), have shorter micro-cycles than the NFL because they compete more frequently. On the other extreme there are endurance athletes that compete once every several weeks or months. It is therefore important to ask ourselves; what are common periodisation practices in this sport and how do they interact with match/competition cadence?
Macro seasonality - while soccer is played for 8 months with a short preseason, American Football environments have dramatically different season durations as well as pre-season characteristics. Tennis and long distance running are even more apart. These elements need to be reflected in the data and modelling for a successful outcome.
An international tournament like no other?
Dynamic contextual factors require constant consideration for athlete management. It can be the difference between winning and losing so-called “unwinnable games”. These factors include the competition calendar, opposition, game times, locations, environmental factors, and team availability. Such contextual fluidity can present a test of our translation abilities. A notable illustration of this is the upcoming 2022 FIFA Men’s World Cup.
For the Member Associations, Qatar presents the usual challenges of an international tournament: travel fatigue and jet lag, where and how to set up their base, training and recovery management during fixture congestion, to name only a few.
Yet, the most noteworthy context is the competition timing. Holding the World Cup in the middle of the (European) season for the first time poses a unique challenge to domestic teams. Which has been explored in more depth by Zone7’s Data Research Analyst Ben Mackenzie using data from other mid-season tournaments, here.
They face continuously shifting contextual factors within their playing squad; in injury availability, training and game loads, fitness-fatigue status, and travel demands. Not to mention the psychological impact of varying performance and success at the tournament.
All factors outside of their control in the international playing group for the time each country remains in the tournament. Additionally, they have to manage training and recovery factors within the playing group that remain with the domestic team.
Thereafter, they have to blend the training and management needs of each athlete, as teams retake the field for the second half of the domestic season. It will be intriguing to watch.
How can AI take into context the changing competition schedule and team-specific approaches to periodisation and recovery?
The success of AI to account for context depends largely on the strategy and inputs from the human operator to aid with contextual appreciation. Practitioners are able to input the team’s competitive schedule, along with their specific periodisation model to the system, for instance. Clearly periodisation context is key to any load management decision.
An example in relation to the aforementioned Qatari World Cup tournament would be for the practitioners at the domestic clubs to provide the system with information around the players that remain under their care.
Factors that could be provided to the AI system include time off, friendly game schedules, altered training micro-cycles, and potential travel demands. All this before potentially adding in any relevant information they may be able to acquire from the international teams about their players competing at the World Cup tournament.
Individual athletes are more than just data points
In our open-access review, led by Dr Stephen West, we illustrated a wide range of contextual factors that influence athlete injury risk and readiness to perform. A large portion of this figure considered athlete level factors.
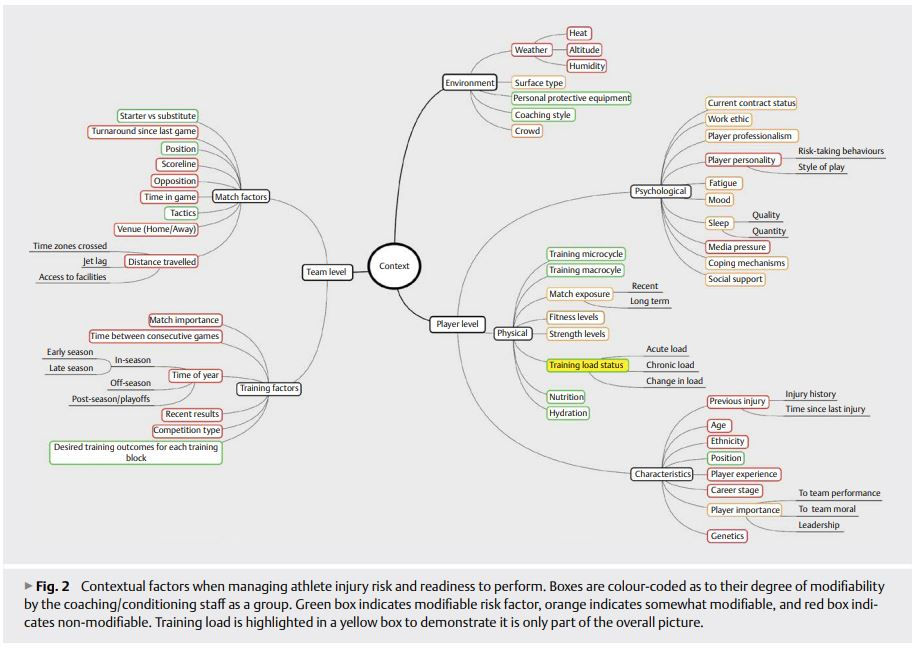
Part of the beauty of sport is the diversity in its competitors. This emphasises the need to embrace individuality. Interestingly, one of the principles of individuality is context, reinforcing how context and individuality are interwoven.
Averages and group level analyses serve their purpose in sports science and analytics. But our analysis is clearly benefitted when context for individual athletes is taken into account.
One of the most valuable contextual factors for athlete management is injury history. It is widely accepted to be one of, if not, the strongest predictor of future injury. Workload management decisions are often different for an older athlete with a history of soft tissue injuries than other athletes, for example.
While prior injuries and age are thought of as non-modifiable factors, research has suggested that increasing risk associated with these factors can be mitigated by eccentric hamstring strength (Opar et al., 2015). As such, connecting sports science data streams, including potential moderators and mediators to workload, is vital to establishing a holistic athlete profile.
Does Zone7’s AI take into context the individual athlete?
Contextualising for the individual athlete is an integral part of how Zone7 model the reality of athletes operating in a highly demanding environment.
Without diving too deeply into Zone7’s intellectual property, they create a holistic athlete profile that connects the dots across as many data points as the environment can provide, including:
Match/competition and training external workload data
Internal workload
Strength assessments
Other environmental and historical
Injury history
This profile is in constant flux and changes every day or even in real-time sometimes. To “use” this profile in a forecasting model and compare one athlete to another, or apply pattern recognition algorithms across a large dataset, a normalisation process occurs. This is sometimes called ‘baselining’ and involves a mathematical analysis to extract an athlete’s baseline, and measure how today’s profile differs from the baseline.
Context as a Double Edged Sword
Clearly, context is an essential ingredient for sports science analysis and interpretation. Yet, I believe it is important to be continually reflective and critical of how we view context. Consider, for example, the following statements:
“He is injury prone”
“She is weak”
“His body doesn’t cope with high training loads”
“They’re a difficult opponent”
Are these representing context? Or bias? When does so-called context actually become bias?
Perhaps context is in danger of declining into bias, when it is a factor that is not, nor cannot be, backed by objective information. The challenge, therefore, is to support our contextual factors with objectivity.
Can we justify the context of “injury prone” with injury history? Can we objectively determine “weak” through strength diagnostics? Do we change our minds if the evidence suggests so?
Much like the need to consider the data input quality, we have a responsibility to be critical of the quality and suitability of the context we add to analytics and AI systems. This responsibility is illustrated in the Netflix documentary, Coded Bias. One summary of the documentary describes how:
“… the very machine-learning algorithms intended to avoid prejudice are only as unbiased as the humans and historical data programming them.”
Thus, it is not the responsibility of the machine. We should be reflective of our own contextual factors, and be wary of when our so-called “context” actually meets bias.
How can a predictive model avoid bias and help practitioners stay away from blind spots?
Bias cannot be detected from within. Once a system is in motion, it is very difficult to detect bias, or drift in accuracy. So the healthy approach in data science is to accept that there will always be bias and to ‘bake’ as many preventive measures into every step of the process as possible.
“We’re blind to our blindness. We have very little idea of how little we know. We’re not designed to know how little we know.” - Daniel Kahneman
It’s important for any system, whether spreadsheet macros or machine learning code, to continuously evaluate accuracy against a solid baseline, much like elite practitioners who reflect, review and evolve their own professional practices. This might take time and effort, whilst also going against what we originally assumed to be true, but is the only way to deepen understanding and create confidence among those relying on these insights.
The Zone7 process of creating forecasting models includes the most rigorous cross validation methods and ongoing monitoring of results, both automatically and by expert operators. In addition, models need refreshing periodically, which again - is a high touch process that must be done with care and the utmost integrity.
In addition, it is key to provide the practitioner with a transparent ‘snapshot’ of the bias risk in the system. Are all incident types detected with the same level of success? How are the current outcomes performing against the established baseline? Only transparency can breed success and positive improvements.
Final Thoughts
As demonstrated by the questions which I received, context is clearly at the forefront of practitioners’ minds. This comes as no surprise. We are acutely aware of the need to consider how various factors, such as the sport, position, individual athlete, and time in season, shape our athlete management decisions. As such, this context must be also injected into any analytics and AI. Responsibility ultimately lies with the practitioner to incorporate appropriate contextual factors, while trying to be mindful of their personal biases.
This article is supported by Zone7. For more information about their technology, visit their website.
Comments