Artificial Intelligence (AI) is fundamentally transforming sports science, potentially offering new ways to enhance performance, prevent injuries, and improve training methodologies. This post provides sports practitioners with an introduction to AI.
What is Artificial Intelligence?
AI involves the development of systems that can perform tasks normally requiring human intelligence, such as learning, decision-making, and problem-solving. In sports science, AI applications range from performance analytics and injury prediction to personalised training programmes and game strategy development.
Machine learning (ML) is increasingly used in sports science research and practice, such as our publication led by Mauro Mandorino that used various ML algorithms to develop an in-situ fitness index of footballers. ML is a subset of AI that develops algorithms by learning from historical data and responding to new data.

Having a basic understanding of these terms is becoming increasingly important for sports scientists as they are becoming more common in research and practice. For a comprehensive understanding of AI concepts and terminology, explore our guide: An AI Dictionary for Sport Scientists.
Practical Considerations in Applying AI in Sports Science
Data Stream Inputs: Garbage In, Garbage Out
The effectiveness of AI in sports science hinges on the quality of data inputs. High-quality, relevant data is crucial for generating reliable insights. Poor data can lead to misleading conclusions, undermining the value of AI applications.
This principle, often summarised as "garbage in, garbage out" (GIGO) emphasises the need for rigorous data collection and management. In relation to sports science, this includes consistent approaches across data managements areas such as:
athlete names
session and drill categorisation
testing protocols
gaps in training load data
removing errors such as velocity spikes
To delve deeper into the importance of data quality, read our article on Big Data: Assessing Sports Science Inputs for AI Modelling.
Trying to manage training load and mitigate injury risk is seen as one of the key applications for AI and ML. While injury history and external load metrics (i.e. from player tracking technologies) are the cornerstone inputs for such projects, it is worth considering how other data streams can be valuable inputs.
In the article Looking Beyond Training Load in Injury Risk Forecasting, I make the case for the importance of other inputs such as internal load, subjective wellness, and objective measures of readiness. These are all vital aspects of an holistic load monitoring system, whether they add objective value to an AI model or not.
Context is Key in Sports Science Analytics
Contextual understanding is pivotal for making meaningful and actionable insights from AI-driven analyses. Without context, data can be ambiguous or even misleading. For instance, physical performance and/or readiness metrics might vary significantly based on factors such as the athlete's current training phase, playing position, match involvement, environmental conditions, or psychological state.
In our publication led by Stephen West, we discussed the wide array of contextual factors that must be considered when managing athlete injury risk and readiness to perform (see Figure below).
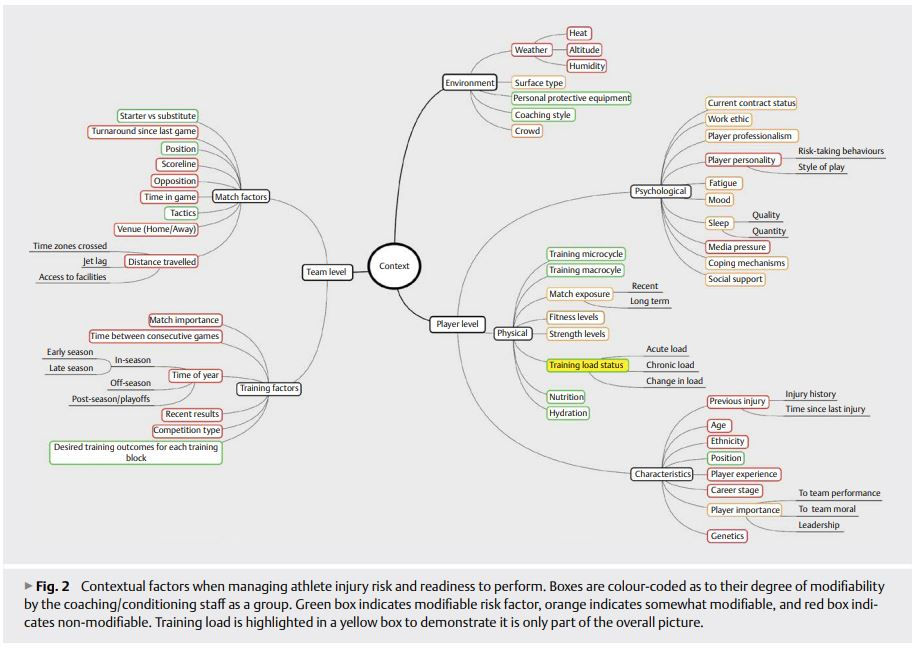
By incorporating these contextual elements, sports scientists can better interpret data and tailor their recommendations accordingly. Explore the necessity of context in sports analytics in our article Why Context Is a Must in Sports Science AI.
Moving from Black Boxes to Interpretable Models
Transparency in AI models is crucial for building trust and ensuring the usability of AI-generated insights. Traditional "black box" models often lack interpretability, making it difficult for practitioners to understand and act upon their outputs. As we discussed in an open access editorial for the British Medical Journal, these are the kinds of potential pitfalls that we need to be cognisant of and responsible for in our roles as ‘Data Stewards’.
Recent advancements in AI focus on creating more interpretable models that provide clearer, actionable insights. This shift towards transparency helps in making AI applications more accessible and effective for sports scientists and coaches. We have previously discussed transparent black boxes with Zone7 to understand the commercial perspective of their approaches.
Extracting More Value from Existing Data
One of the significant benefits of AI is its ability to extract new insights from the data already being collected. By applying advanced ML techniques, AI can uncover patterns and correlations that might not be evident through traditional analysis methods. This could be crucial given the complexity of injury risk and performance.
For example, in our recent study, ML was used to predict soccer players' fitness status based on in-situ training data, demonstrating its potential to enhance decision-making in sports. This application is another example of ‘invisible monitoring’, in which we strive to gain deeper insights from data already collected, eliminating the need for additional testing. AI could be key to unlocking these insights and reducing data collection burden on our athletes.
Designing Effective AI Outputs, Reports and Visualisations
AI outputs must be designed to be clear and actionable to avoid overwhelming users with complex or ambiguous information. Effective design of AI outputs ensures that the insights provided are easily interpretable and can be directly applied to improve training and performance. This involves presenting data in a user-friendly format and providing context-specific recommendations.
Conversely, when poorly communicated, AI outputs can confuse the end user. Probably the most famous case of this was Gary Kasparov’s confusion as a result of a unusual chess move by IBM’s AI-driven Deep Blue. Read more about this story and designing explainable AI outputs in Designing Explainable AI Outputs in Sports Science.
Supporting a Proactive and Hybrid Approach
Training management is already moving from a reactive approach to one that is more proactive, enabling practitioners to anticipate and address issues before they become problematic. This includes training session and drill planning, with the use of a drill database and forecasting training load outcomes, as well as associated injury risk forecasts. AI has the potential to further improve this.
However, we should never be blindly guided by machines. A hybrid approach involves integrating AI insights with human expertise that leverages the strengths of both. AI can help identify potential injury risks, optimise training loads, and personalise training programmes, while human practitioners can provide the necessary contextual understanding and adjust strategies based on real-time observations. This is discussed further in the article: Man and Machine: A Hybrid Approach to Athlete Load Management.
Using AI Tools for Sports Science Research
Learning how to utilise AI will not only save practitioners time, but will potentially set them apart in job interviews and roles. It is about learning how to leverage them. As my friend Carlos Balsalobre argued, people will still be needed to ask the right questions and be critical of the answers.
AI tools can significantly assist sports science practitioners in their daily roles, from research to practical applications. Tools like ChatGPT can be invaluable for conducting literature reviews, generating research ideas, and drafting reports. Many of these tools are based on large language models (LLM), which learn from large datasets and generate text in response to prompts.
By automating routine tasks, these tools free up practitioners to focus on more strategic activities and analyses.
Discover how AI tools can enhance your research capabilities with these guides:
FAQs on AI in Sports Science
What is Artificial Intelligence?
Artificial Intelligence (AI) is the ability of a digital computer or computer-controlled robot to perform tasks commonly associated with intelligent beings. The term is frequently applied to the project of developing systems endowed with the intellectual processes characteristic of humans, such as the ability to reason, discover meaning, generalise, or learn from past experience. For more on AI terminology, see our AI Dictionary for Sports Scientists.
How is AI used in sports science?
AI in sports science involves using statistical techniques based on AI to enhance various aspects of sports, such as performance analysis, injury prevention, training optimisation, and game strategy development. It leverages machine learning, data analytics, and other AI tools to provide deeper insights and more effective solutions for athletes and coaches.
How can AI improve injury prevention in sports?
Injury prevention is a contentious topic in sports science. AI has shown the potential to assist with injury risk mitigation by analysing large datasets to identify patterns and risk factors associated with injuries.
What are the challenges of using AI in sports science?
The main challenges include ensuring high-quality data inputs, maintaining transparency and interpretability of AI models, and integrating AI seamlessly into existing workflows. It's crucial to combine AI insights with human expertise to address these challenges effectively.
How can practitioners use AI tools in their roles?
Practitioners can use AI tools for various tasks such as conducting literature reviews, generating research ideas, and drafting reports. These tools help automate routine tasks, allowing practitioners to focus on strategic and high-impact activities. For practical applications, see our guides on How to Use ChatGPT for Sports Science Research and An Introduction to ChatGPT Plus for Sports Science.
Comments